Chemical Computing: Bridging Chemistry and Digital Innovation
Emphasizing its revolutionary potential, present advances, and future possibilities in transforming technology and improving computational skills, this paper investigates the concepts, methods, and applications of chemical computing.
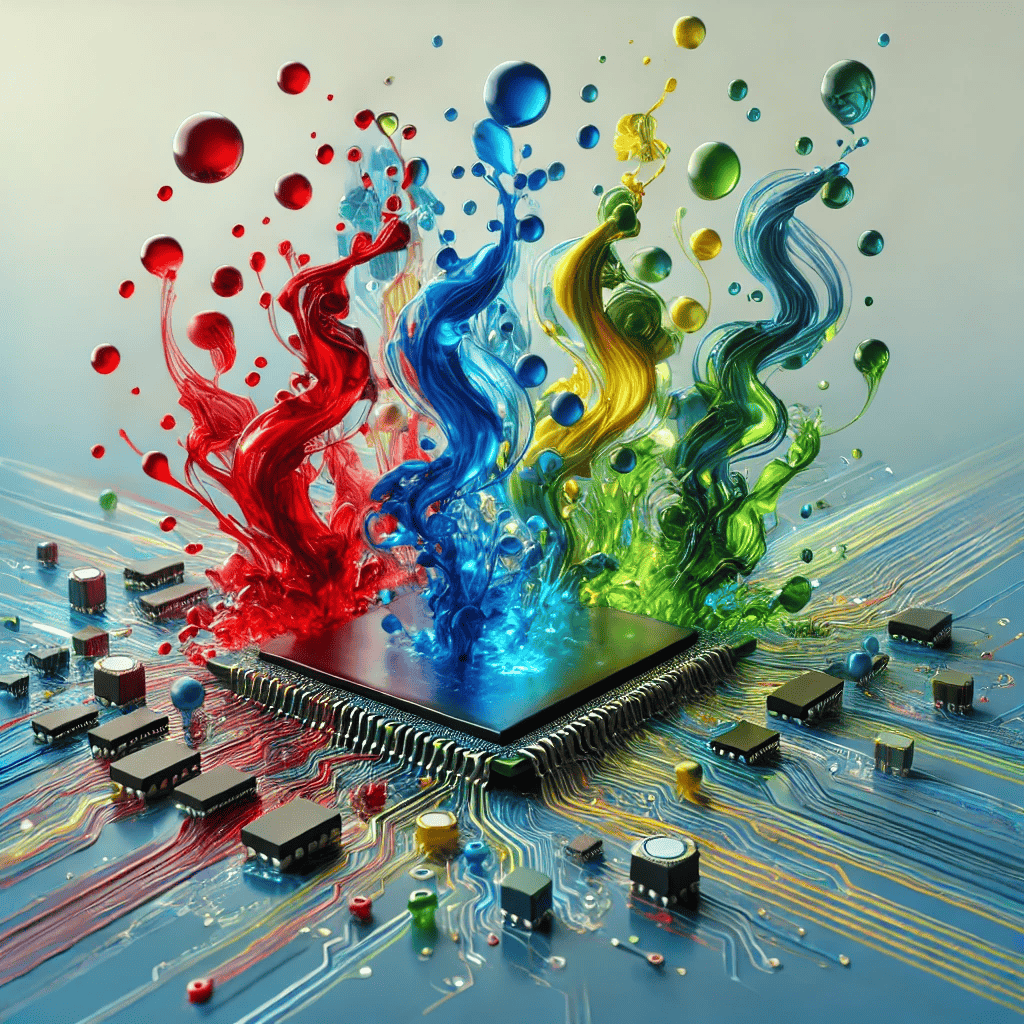
Introduction
At the junction of chemistry and computer science, chemical computing is the application of chemical reactions and methods to carry out computational chores. Important progress in this field has been achieved over years. Chemical analysis first automated early in 1914 (Tan et al., 2020), and since then, chemical synthesis has extensively embraced automated methods. Utilizing enzyme-biocatalyzed processes, the idea of biocomputing has evolved allowing logic processing of biochemical signals for integrating electronic and biological systems (Katz, 2012).
Modern technology clearly shows the relevance of chemical computation. Common logic operations can today be replicated by molecular devices grounded in chemical techniques (Pischel, 2007). Development of computational methods for scaffold generation and analysis has affected medicinal chemistry by means of impact on scaffold development and analysis. Computational expressivity of chemical reaction networks has been demonstrated, hence allowing error-free computing (Brijder et al., 2017). Moreover, formalized deterministic function computing with chemical reaction networks offers a paradigm for chemistry in well-mixed fluids (Chen et al., 2012).
Chemical computing has quite many possible uses. It provides a technique to close the distance between electrical and biological systems (Katz, 2012). By means of a chemical ontology, automated compound classification helps to translate expert chemistry knowledge into computer-interpretable forms, hence facilitating compound assignments and categorization (Bobach et al., 2012). Furthermore investigated is the computational complexity of atomic chemical reaction networks, thereby highlighting their capacity to calculate predicates and functions (Doty & Zhu, 2018).
Chemical computing has developed greatly and provides a special kind of computation by using chemical reactions. Its possibilities in many disciplines are shown by its uses from biocomputing to automatic compound classification. The integration of chemical computing into many spheres is probably going to increase as technology develops, thereby creating fresh research and invention opportunities.
Core Concepts and Mechanisms
The fundamental ideas of chemical computation are the use of chemical reactions and methods to accomplish computer tasks. Unlike conventional electronic computing techniques dependent on electrical signals, chemical computation functions within geometrically-constrained excitable chemical media. Changes in reagent concentrations are employed in these media to transmit information and enable computation (Sun & Zhao, 2013). Especially, chemical computing uses reaction-diffusion processes—best shown by the Belousov-Zhabotinsky reaction—which may run low-power, massive parallel computations (Fyrigos et al., 2019). Moreover, scientists have investigated employing chemical flow systems and bistable reactions logic gates and finite-state machines, therefore illustrating the possibility to solve complex computing problems via chemical approaches (Katz & Bocharova, 2012).
Unlike electronic systems able to create complex interconnected networks, chemical computing systems usually operate as individual logic gates and cannot be connected into networks when compared with conventional electronic computing methods (Katz & Privman, 2010). Nevertheless, by bridging the gap between electronic and biological systems and enabling creative applications in integrating biological and electronic components, chemical computing has unique benefits (Katz, 2012). Furthermore, chemical computing devices can use reaction-diffusion processes to run extremely sophisticated computational tasks, therefore displaying a different computational strategy than conventional electronic approaches (Rambidi & Yakovenchuk, 2001).
Using chemical reactions and procedures, chemical computation presents a fresh perspective for completing computational chores. Chemical computing offers special capabilities and possible uses across many fields, therefore stressing its relevance in improving computational technologies even if it deviates from conventional electrical computing approaches in operational principles and limits.
Applications and Future Prospects
Chemical computing’s adaptability and possible influence are shown by current useful applications across many disciplines. Automation using microfluidics-assisted chemical synthesis and artificial intelligence systems is transforming the drug discovery process and increasing efficiency and creativity. Schneider 2017. Moreover, the use of machine learning techniques to forecast more selective catalysts shows the possibility to speed up material discovery (Zahrt et al., 2019). Accelerating chemical kinetics computations can improve climate models and find use in several scientific disciplines in the domain of atmospheric research (Alvanos & Christoudia, 2019). Furthermore stressing the influence on sustainable energy solutions, developments in predictive chemistry allow rational design techniques for biofuels with better qualities (Lockwood et al., 2022).
Looking ahead, chemical computing offers possibilities as well as problems. Though they present difficulties in their implementation and scalability, quantum computing platforms promise for cryptography, optimization, and machine learning applications (Tamrakar, 2019). Deep learning in protein structure modeling is driving a scientific revolution influencing disciplines outside of biology and chemistry (Gao et al., 2020). Blockchain technology’s inclusion into computer paradigms presents distributed and safe solutions with general uses (Mendis et al., 2021). Still unresolved are issues include the limits of present technologies and the need of developments in modeling to precisely forecast reaction outcomes (Mohan et al., 2005; Dewyer et al., 2017).
Chemical computing has a major influence on society, research, and technology. Chemical computing is driving invention in many different fields, from improving drug discovery techniques to allowing sustainable energy alternatives. Its transforming power is highlighted by our capacity to speed material discovery, improve climate models, and modify biofuel design. The integration of chemical computing into many applications is poised to transform sectors and scientific research as hurdles are solved and innovations keep on. This will open the path for a day when computational chemistry takes front stage in tackling difficult problems.
References
Bobach, C., Böhme, T., Laube, U., Püschel, A., & Weber, L. (2012). Automated compound classification using a chemical ontology. Journal of Cheminformatics, 4(1). https://doi.org/10.1186/1758-2946-4-40
Brijder, R., Doty, D., & Soloveichik, D. (2017). Democratic, existential, and consensus-based output conventions in stable computation by chemical reaction networks. Natural Computing, 17(1), 97-108. https://doi.org/10.1007/s11047-017-9648-8
Chen, H., Doty, D., & Soloveichik, D. (2012). Deterministic function computation with chemical reaction networks., 25-42. https://doi.org/10.1007/978-3-642-32208-2_3
Doty, D. and Zhu, S. (2018). Computational complexity of atomic chemical reaction networks. Natural Computing, 17(4), 677-691. https://doi.org/10.1007/s11047-018-9687-9
Hu, Y., Stumpfe, D., & Bajorath, J. (2016). Computational exploration of molecular scaffolds in medicinal chemistry. Journal of Medicinal Chemistry, 59(9), 4062-4076. https://doi.org/10.1021/acs.jmedchem.5b01746
Katz, E. (2012). Bioelectronic devices controlled by enzyme‐based information processing systems., 61-80. https://doi.org/10.1002/9783527645480.ch4
Pischel, U. (2007). Chemical approaches to molecular logic elements for addition and subtraction. Angewandte Chemie, 46(22), 4026-4040. https://doi.org/10.1002/anie.200603990
Tan, S., Naraharisetti, P., Chin, S., & Lee, L. (2020). Simple visual-aided automated titration using the python programming language. Journal of Chemical Education, 97(3), 850-854. https://doi.org/10.1021/acs.jchemed.9b00802
Fyrigos, I., Ntinas, V., Sirakoulis, G., Adamatzky, A., Erokhin, V., & Rubio, A. (2019). Wave computing with passive memristive networks.. https://doi.org/10.1109/iscas.2019.8702789
Katz, E. and Bocharova, V. (2012). Molecular information processing: from single molecules to supramolecular systems and interfaces – from algorithms to devices – editorial introduction., 1-9. https://doi.org/10.1002/9783527645442.ch1
Katz, E. and Privman, V. (2010). Enzyme-based logic systems for information processing. Chemical Society Reviews, 39(5), 1835. https://doi.org/10.1039/b806038j
Rambidi, N. and Yakovenchuk, D. (2001). Chemical reaction-diffusion implementation of finding the shortest paths in a labyrinth. Physical Review E, 63(2). https://doi.org/10.1103/physreve.63.026607
Sun, M. and Zhao, X. (2013). Multi-bit binary decoder based on belousov-zhabotinsky reaction. The Journal of Chemical Physics, 138(11). https://doi.org/10.1063/1.4794995
Alvanos, M. and Christoudias, T. (2019). Accelerating atmospheric chemical kinetics for climate simulations. Ieee Transactions on Parallel and Distributed Systems, 30(11), 2396-2407. https://doi.org/10.1109/tpds.2019.2918798
Dewyer, A., Argüelles, A., & Zimmerman, P. (2017). Methods for exploring reaction space in molecular systems. Wiley Interdisciplinary Reviews Computational Molecular Science, 8(2). https://doi.org/10.1002/wcms.1354
Gao, W., Mahajan, S., Sulam, J., & Gray, J. (2020). Deep learning in protein structural modeling and design. Patterns, 1(9), 100142. https://doi.org/10.1016/j.patter.2020.100142
Lockwood, K., Ahmed, S., Huq, N., Stutzman, S., Foust, T., & Labbe, N. (2022). Advances in predictive chemistry enable a multi-scale rational design approach for biofuels with advantaged properties. Sustainable Energy & Fuels, 6(23), 5371-5383. https://doi.org/10.1039/d2se00773h
Mendis, G., Wu, Y., Jin, W., Sabounchi, M., & Roche, R. (2021). A blockchain-powered decentralized and secure computing paradigm. Ieee Transactions on Emerging Topics in Computing, 9(4), 2201-2222. https://doi.org/10.1109/tetc.2020.2983007
Mohan, V., Gibbs, A., Cummings, M., Jaeger, E., & DesJarlais, R. (2005). Docking: successes and challenges. Current Pharmaceutical Design, 11(3), 323-333. https://doi.org/10.2174/1381612053382106
Schneider, G. (2017). Automating drug discovery. Nature Reviews Cancer, 17(2), 97-113. https://doi.org/10.1038/nrd.2017.232
Tamrakar, A. (2019). Quantum computing: a comprehensive review. Turkish Journal of Computer and Mathematics Education (Turcomat), 10(3), 1634-1642. https://doi.org/10.61841/turcomat.v10i3.14623
Zahrt, A., Henle, J., Rose, B., Wang, Y., Darrow, W., & Denmark, S. (2019). Prediction of higher-selectivity catalysts by computer-driven workflow and machine learning. Science, 363(6424). https://doi.org/10.1126/science.aau5631